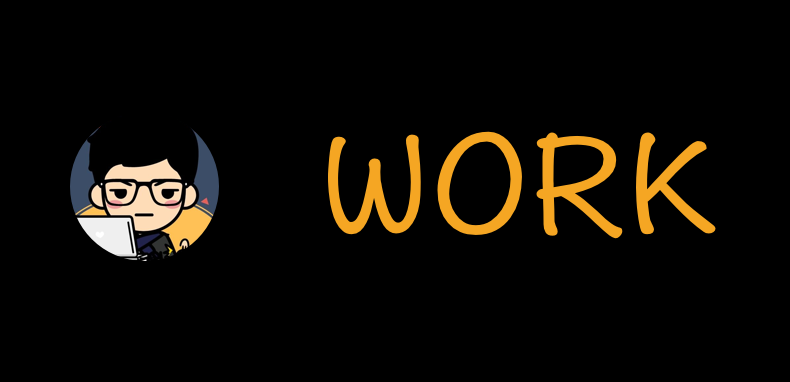
BloomFilter 实现原理及使用
布隆过滤器介绍
布隆过滤器(Bloom Filter)由 Burton Howard Bloom
在 1970 年提出,是一种空间效率高的概率型数据结构。它专门用来检测集合中是否存在特定的元素。其实对于判断集合中是否存在某个元素,我们平时都会直接使用比较算法,例如:
- 如果集合用线性表存储,查找的时间复杂度为 O(n);
- 如果用平衡 BST(如 AVL树、红黑树)存储,时间复杂度为 O(logn);
- 如果用哈希表存储,并用链地址法与平衡 BST 解决哈希冲突(参考 JDK8 的 HashMap 实现方法),时间复杂度也要有O[log(n/m)],m 为哈希分桶数。
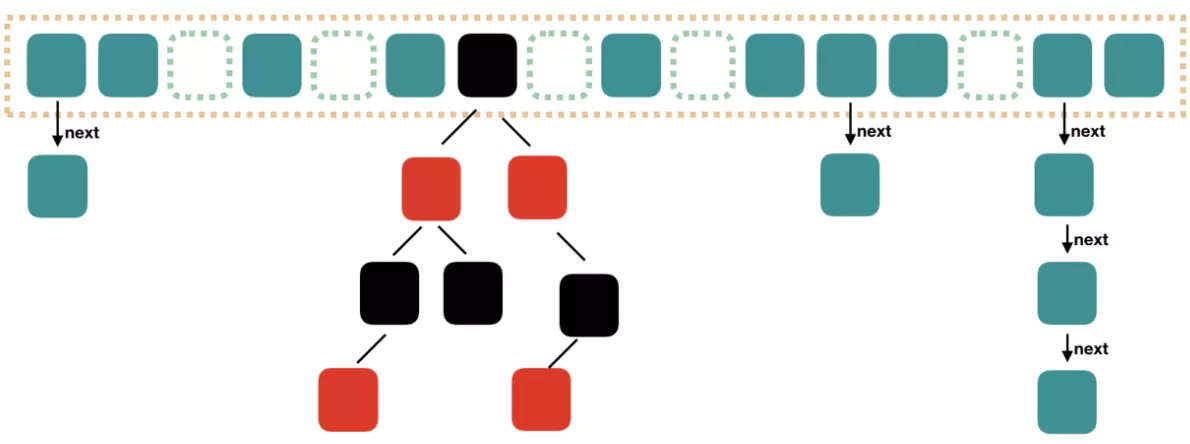
如果采用上面提到的一些方法,需要将实际数据都要存储到集合中,才能真正判断元素是否存在,会占用很大的内存空间,而且对于上面计算的时间复杂度,如果集合中元素非常多时,查找效率并不高。Bloom Filter 就是为了解决这些问题应运而生的。
Bloom Filter 设计思想
Bloom Filter 是由一个长度为 m 的比特位数组(bit array)与 k 个哈希函数(hash function)组成的数据结构。位数组均初始化为 0,所有哈希函数都可以分别把输入数据尽量均匀地散列。
当要插入一个元素时,将其数据分别输入 k 个哈希函数,产生 k 个哈希值。以哈希值作为位数组中的下标,将所有 k 个对应的比特置为 1。
当要查询(即判断是否存在)一个元素时,同样将其数据输入哈希函数,然后检查对应的 k 个比特。如果有任意一个比特为 0,表明该元素一定不在集合中。如果所有比特均为 1,表明该元素有(较大的)可能性在集合中。为什么不是一定在集合中呢?因为一个比特被置为 1 有可能会受到其他元素的影响,这就是所谓“假阳性”(false positive)。相对地,“假阴性”(false negative)在 Bloom Filter 中是绝不会出现的。
下图示出一个 m=18, k=3 的 Bloom Filter 示例。集合中的 x、y、z 三个元素通过 3 个不同的哈希函数散列到位数组中。当查询元素 w 时,因为有一个比特为0,因此 w 不在该集合中。
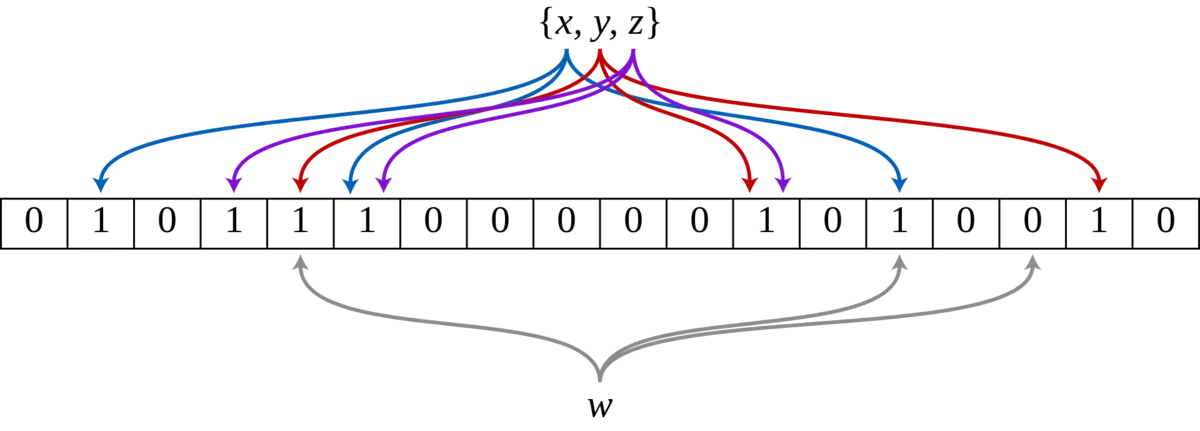
Bloom Filter 的优缺点与用途
优点:
- 不需要存储数据本身,只用比特表示,因此空间占用相对于传统方式有巨大的优势,并且能够保密数据;
- 时间效率也较高,插入和查询的时间复杂度均为O(k);
- 哈希函数之间相互独立,可以在硬件指令层面并行计算。
缺点:
- 存在假阳性的概率,不适用于任何要求 100% 准确率的场景;
- 只能插入和查询元素,不能删除元素,这与产生假阳性的原因是相同的。我们可以简单地想到通过计数(即将一个比特扩展为计数值)来记录元素数,但仍然无法保证删除的元素一定在集合中。
所以,Bloom Filter 在对查准度要求没有那么苛刻,而对时间、空间效率要求较高的场合非常合适,本文第一句话提到的用途即属于此类。另外,由于它不存在 假阴性 问题,所以用作“不存在”逻辑的处理时有奇效,比如可以用来作为 缓存系统(如Redis)的缓冲,防止缓存穿透。
Google Guava 中 Bloom Filter 的使用
A Bloom filter offers an approximate containment test with one-sided error: if it claims that an element is contained in it, this might be in error, but if it claims that an element is not contained in it, then this is definitely true.
Bloom filter 提供了一个单方面错误的近似包含测试:如果它声称某个元素包含在其中,则这可能是错误的(可能不包含在其中);但是如果它声称某个元素不包含在其中,那这一定是正确的(一定不包含在其中)。
1 | import com.google.common.hash.BloomFilter; |
Redis 中 Bloom Filter 的使用
Redis 中使用 BloomFilter 需要安装 RedisBloom 插件,下载源码编译后生成一个 rebloom.so
文件,然后需要在在 Redis 的配置文件 redis.conf
中加入该模块即可:
1 | loadmodule /${path}/rebloom.so |
1 | bf.add test 1 |
关于 RedisBloom
的详细说明可以参考文档:RedisBloom - Probabilistic Datatypes Module for Redis
Redis BloomFilter 在 java 中的应用,可以使用 jrebloom-${version}.jar
jar 包中提供的功能:
1 | <dependency> |
1 | import io.rebloom.client.Client; |
参考资料
- Bloom Filters by Example
- 布隆过滤器(Bloom Filter)原理及 Guava 中的具体实现
- RedisBloom - Probabilistic Datatypes Module for Redis
- 详细解析Redis中的布隆过滤器及其应用
- 帮你解读什么是Redis缓存穿透和缓存雪崩(包含解决方案)
- Redis安装布隆过滤器插件 bloomfilter
- 通俗易懂讲布隆过滤器
- 布隆过滤器究竟是什么,这篇讲的明明白白的!